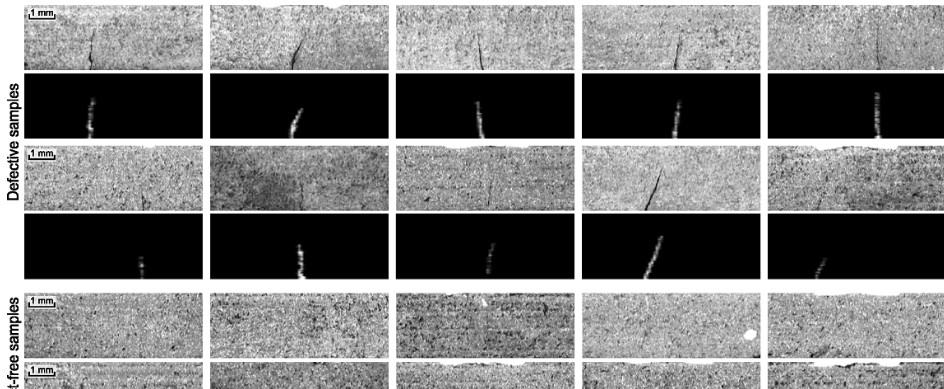
Surface Defect Detection
Constantly summarizing open source dataset and important critical papers in the field of surface defect research are very important. š

Contributors
Surface Defect Detection is all of us
Our contributors 1
Thank you for supporting Surface Defect Detection.

About

Introduction
At present, surface defect equipment based on machine vision has widely replaced artificial visual inspection in various industrial fields, including 3C, automobiles, home appliances, machinery manufacturing, semiconductors and electronics, chemical, pharmaceutical, aerospace, light industry and other industries. Traditional surface defect detection methods based on machine vision often use conventional image processing algorithms or artificially designed features plus classifiers. Generally speaking, imaging schemes are usually designed by using the different properties of the inspected surface or defects. A reasonable imaging scheme helps to obtain images with uniform illumination and clearly reflect the surface defects of the object. In recent years, many defect detection methods based on deep learning have also been widely used in various industrial scenarios.
Compared with the clear classification, detection and segmentation tasks in computer vision, the requirements for defect detection are very general. In fact, its requirements can be divided into three different levels: "what is the defect" (classification), "where is the defect" (positioning) And "How many defects are" (split).
Table of Contents
- [Introduction](#introduction)
- [Key Issues](#1-key-issues-in-surface-defect-detection)
Ā - [Small Sample Problem](#1small-sample-problem)
Ā - [Real-time Problem](#2real-time-problem)
- [Common Datasets](#2-common-datasets-for-industrial-surface-defect-detection)
Ā - [Steel Surface: NEU-CLS](#1steel-surface-neu-cls)
Ā - [Solar Panels: elpv-dataset](#2solar-panels-elpv-dataset)
Ā - [Metal Surface: KolektorSDD](#3metal-surface-kolektorsdd)
Ā - [PCB Inspection: DeepPCB](#4pcb-inspection-deeppcb)
Ā - [Fabric Defects Dataset: AITEX](#5fabric-defects-dataset-aitex)
Ā - [Fabric Defect Dataset (Tianchi)](#6fabric-defect-dataset-tianchi)
Ā - [Aluminium Profile Surface Defect Datasetļ¼Tianchiļ¼](#7aluminium-profile-surface-defect-datasettianchi)
Ā - [Weakly Supervised Learning for Industrial Optical Inspectionļ¼DAGM 2007ļ¼](#8weakly-supervised-learning-for-industrial-optical-inspectiondagm-2007)
Ā - [Cracks on the Surface of Construction](#9cracks-on-the-surface-of-the-construction)
Ā - [Magnetic Tile Dataset](#10magnetic-tile-dataset)
Ā - [RSDDs: Rail Surface Defect Datasets](#11rsdds-rail-surface-defect-datasets)
Ā - [Kylberg Texture Dataset v.1.0](#12kylberg-texture-dataset-v10)
- [Papers](#3-surface-defect-detection-papers)
- [Acknowledgements](#acknowledgements)
- [Download](#download)
- [Notification](#notification)
- [Community](#-community)
Go to web --> https://github.com/Charmve/Surface-Defect-Detection
At present, surface defect equipment based on machine vision has widely replaced artificial visual inspection in various industrial fields, including 3C, automobiles, home appliances, machinery manufacturing, semiconductors and electronics, chemical, pharmaceutical, aerospace, light industry and other industries. Traditional surface defect detection methods based on machine vision often use conventional image processing algorithms or artificially designed features plus classifiers. Generally speaking, imaging schemes are usually designed by using the different properties of the inspected surface or defects. A reasonable imaging scheme helps to obtain images with uniform illumination and clearly reflect the surface defects of the object. In recent years, many defect detection methods based on deep learning have also been widely used in various industrial scenarios.
Compared with the clear classification, detection and segmentation tasks in computer vision, the requirements for defect detection are very general. In fact, its requirements can be divided into three different levels: "what is the defect" (classification), "where is the defect" (positioning) And "How many defects are" (split).
Table of Contents
- [Introduction](#introduction)
- [Key Issues](#1-key-issues-in-surface-defect-detection)
Ā - [Small Sample Problem](#1small-sample-problem)
Ā - [Real-time Problem](#2real-time-problem)
- [Common Datasets](#2-common-datasets-for-industrial-surface-defect-detection)
Ā - [Steel Surface: NEU-CLS](#1steel-surface-neu-cls)
Ā - [Solar Panels: elpv-dataset](#2solar-panels-elpv-dataset)
Ā - [Metal Surface: KolektorSDD](#3metal-surface-kolektorsdd)
Ā - [PCB Inspection: DeepPCB](#4pcb-inspection-deeppcb)
Ā - [Fabric Defects Dataset: AITEX](#5fabric-defects-dataset-aitex)
Ā - [Fabric Defect Dataset (Tianchi)](#6fabric-defect-dataset-tianchi)
Ā - [Aluminium Profile Surface Defect Datasetļ¼Tianchiļ¼](#7aluminium-profile-surface-defect-datasettianchi)
Ā - [Weakly Supervised Learning for Industrial Optical Inspectionļ¼DAGM 2007ļ¼](#8weakly-supervised-learning-for-industrial-optical-inspectiondagm-2007)
Ā - [Cracks on the Surface of Construction](#9cracks-on-the-surface-of-the-construction)
Ā - [Magnetic Tile Dataset](#10magnetic-tile-dataset)
Ā - [RSDDs: Rail Surface Defect Datasets](#11rsdds-rail-surface-defect-datasets)
Ā - [Kylberg Texture Dataset v.1.0](#12kylberg-texture-dataset-v10)
- [Papers](#3-surface-defect-detection-papers)
- [Acknowledgements](#acknowledgements)
- [Download](#download)
- [Notification](#notification)
- [Community](#-community)
Go to web --> https://github.com/Charmve/Surface-Defect-Detection